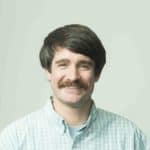
Todd Whitehead
January 25, 2024
Coaches love team rankings! They want to know how their team stacks up against other teams in their league in the statistical categories that matter most to winning – offensive and defensive efficiency, field goal percentage, turnover rate, etc. But they also want to be compared to their rivals fairly, in an apples-to-apples kind of way. The trouble is, not every team faces the same challenges throughout a season. In amateur basketball, in particular, the level of competition can vary widely from one team to the next and over the course of the season, too.
To help level the playing field, we are adding a new leaderboard to the Synergy site that displays a team’s stats after adjusting for the following factors:
-Strength of opponents,
-Venue (home or away),
-Rest (travel schedule),
-Blowouts (downplaying the importance of “garbage time”), and
-Hot streaks (or “what have you done for me lately?”).
With the new Team Adjusted Leaderboard, coaches will be better equipped to answer some of the most fundamental questions of team evaluation: Where does our performance rank compared to the rest of the league? Is our performance getting better or worse? And what should our performance expectations be going forward?
Most of the team stats featured on the new adjusted leaderboard will be familiar to Synergy customers who have used our “unadjusted” leaderboards before – you’ll see the adjusted versions of stats like points per possession (PPP), field goal percentage (FG%), effective field goal percentage (eFG%), Synergy Shot Quality (SSQ), 3-point field goal percentage (3 FG%), turnover rate (TO%), and free throw rate (%FT) for a team’s offense and defense – but there will also be one new metric included, the Synergy Net Rating (SNR). SNR represents a team’s expected margin of victory in entirely neutral conditions: Playing against an average team on a neutral court with no rest advantage in a 100-possession game, similar to KenPom’s adjusted expected margin (AdjEM) or the NCAA’s NET ranking. Because team rating metrics like these are presented on a per-100 possession scale, SNR is roughly the equivalent of 100 times the difference between a team’s offensive and defensive PPP stats, so SNR ~ 100 * (OFF PPP – DEF PPP).
For example, here are the 2023 end-of-year Synergy Net Ratings for the 12 WNBA teams.
The Las Vegas Aces had an adjusted offensive efficiency of 1.02 points per possession and an adjusted defensive efficiency of 0.91 points per possession (which is a difference of about +0.11 PPP) and a corresponding SNR of +12.9 points per 100 possessions. This means that, if the Aces played an average WNBA team on a neutral court in a 100-possession game, we would expect them to win by about 13 points. In the last decade, no WNBA team has finished the season with a higher SNR than the Aces this year. Conveniently, they also won the WNBA title. So, it looks like the rankings are working!
Let’s break down each factor that goes into the calculation, one by one, so we can see where these SNR numbers come from.
It’s no secret that the strength of a team’s opponents can have a huge impact on their win-loss record. And because each team has tougher and easier parts of its schedule, a team’s record can be a poor representation of its true strength at various times during the season. Take Creighton’s 2022-23 results, for example.
Creighton started the season with games against four unranked teams who finished the year outside the SNR top 150 with an average SNR of -8.3. To that point in the season, Creighton had the 343rd most-difficult strength of schedule in Division 1 (out of 362 teams). That was the easiest four-game stretch of Creighton’s season, and they won those games by a total of 105 points, climbing to no. 10 in the AP poll in the process (and eventually reaching as high as no. 7).
The Bluejays boomeranged their way from that mellow warmup stretch immediately into the most grueling eight-game sprint of their schedule as they faced four straight ranked opponents (Texas Tech, Arkansas, Arizona, and Texas), before taking on three more stiff out-of-conference challengers (Nebraska, BYU, and Arizona State) and then squaring off with their first Big East rival of the season (Marquette). All eight of those foes finished the year in the SNR top 100 with an average SNR of +16.8 (and three of those teams finished in the SNR top 20).
The result? Creighton went 2-6 during that stretch. They lost six straight games, dropping to 0.500 on the year and crashing their way out of the AP Top 25. It must have felt like a real rollercoaster for the Bluejays! But the truth is, Creighton was probably a little overrated after winning their first six games and a little underrated after losing their next six, at least in the eyes of the AP voters.
In contrast, because SNR accounts for opponent strength as part of the rating’s math, it’s not as easily fooled by the results of a stretch of easy or tough games. Thus, Creighton’s SNR kept a more even keel over their first 12 games than their rocky win-loss record did.
All in all, Creighton played a very tough schedule last year – the 16th most-difficult slate in the country by our estimation – losing a dozen times before the tournament. Still, SNR had them 14th on the day of the selection show. And, despite being saddled with a 6 seed (a seeding more in line with their AP rank than their SNR rank), they showed their quality by making a run into the Elite 8 where they battled the eventual runners-up, San Diego State, to the wire, missing out on a trip to the Final 4 by just one free throw.
In assigning credit for wins and losses, our team strength metric accounts for venue – weighing whether a team’s games were played at home or on the road in tallying up their results. Home court advantage differs across leagues, divisions, and seasons, but nearly every team is a few points better at home than they are on the road, with the average home-court advantage in, e.g., NCAA Division 1 men’s basketball being worth somewhere around 4 extra points per 100 possessions. Not every college gym is created equal, though. Some teams have bigger home court advantages than others, with the biggest bonus in college basketball being 1 point more than average (nearly +5 HCA) and the smallest being 1 point below average (but still about +3 HCA).
A look at the list of schools with the strongest home court advantages over the past ten years reveals a few themes about who has the biggest edge at home.
Like the NBA’s Denver Nuggets, college teams who play at elevation enjoy a physiological advantage over their opponents. Games played above 4,000 feet leave opponents gasping for thin air, a phenomenon which has helped several high-altitude teams to establish above-average home court advantages, including Colorado (5430’), Utah (4265’), and Utah State (4534’) who are each in the Top 10 for biggest HCA according to our model.
Of course, crowd noise is synonymous with home court advantage and many of the schools with the highest attendance are also at the top of the HCA list. Purdue (14,804 fans per game), Louisville (13,242), and Arkansas (19,022) were all in the Top 30 for average attendance last season and each was also among the Top 20 strongest home court advantages. West Virginia, drawing over 10,000 rugged Mountaineer fanatics each game, had the biggest advantage over the last ten years.
Some other more renowned venues also fared quite well on our statistically-derived list of top home court advantages – with famous gyms like Cameron Indoor Stadium, Allen Fieldhouse, and Pauley Pavilion all landing in the 20s and Indiana’s Assembly Hall checking in at no. 9.
It’s worth noting that, because “home court advantage” boils down to the difference between home- and road-game performance, we could be pessimistically re-brand it as “road court disadvantage” without changing the math at all. Teams with relatively small home court advantages could also be described as teams with relatively strong road records. This may help explain why some venues – dripping with tradition and mystique though they may be – didn’t find themselves even higher on our list.
Finally, one other team in the Top 5 for home court advantage who we haven’t mentioned yet: Oregon. Their gym isn’t at the top of a mountain summit, and they don’t draw enormous crowds. So, what is giving Oregon that extra 0.7-point boost? My hunch is it’s the court.
In stacking up the wins and losses, our team strength metric also accounts for rest advantages (or disadvantages) caused by the inevitable idiosyncrasies of scheduling. These scheduling challenges can have a big impact, especially in the NBA. It’s well known that teams who are playing in the second game of back-to-back games tend to underperform. In matchups where one team has had a few nights off and the other team is playing for the second night in a row, the well-rested team wins more often than would be expected based on win-loss records alone. And when a road trip stretches out for multiple weeks, like the Spurs infamous Rodeo Roadtrip, the visiting team’s performance tends to suffer.
Crucially – due to the serious logistical challenges of scheduling 1230 regular-season games for 30 teams across 28 cities while avoiding concerts and hockey games and all the other arena commitments that the schedule makers must accommodate – these types of rest disadvantages aren’t necessarily distributed equitably around the league. Teams can have difficult stretches of weeks (or even longer) during which their travel and rest difficulties are more challenging than the situations their competitors are facing.
Our team strength metric accounts for these rest disadvantages – giving a little extra credit to the teams who have been able to perform well despite being faced with challenging travel situations. And these adjustments are made in the SNR calculations for all leagues and levels (not just the NBA).
The model that we are using to adjust team stats de-emphasizes possessions that happen in “garbage time.” During these possessions, when the outcome of the game feels like a foregone conclusion, teams are less likely to be playing their best players and one (or both) teams may experience lapses in focus or motivation. As a result, a garbage time possession tells us less about the true strength of a team than a typical possession would. Still, we don’t want to completely discount the stats teams are producing in these low-leverage moments either, because they aren’t entirely meaningless.
And, anyways, determining when garbage time has begun isn’t an exact science. Even before you consider that each coach will have his or her own philosophy about throwing in the towel and that each team will have its own mentality towards accepting defeat, you need to remember that each league also has different rules surrounding game- and shot-clock lengths and end-of-game fouling situations. So, with all the leagues and levels that Synergy covers, it can get a little complicated!
One thing that is clear is that the chances of a team making a comeback in various situations of time and score will vary by league. For example, a team who is trailing by 10 points with 9 minutes left in an NBA game is in a tenuous but not insurmountable position. Over the last five years, NBA teams who have found themselves in this situation have mounted a successful comeback 11% of the time whereas college women’s teams have had about half as much success overcoming that same type of deficit (just a 6% comeback rate). Because the probability of making a comeback varies by league, it follows that a coach’s approach to conceding defeat will also vary by league.
We are using a data-driven approach to help us decide how to best weight possessions from different points in the game on a league-by-league basis using historical results to identify the approach that will optimize our ability to predict future outcomes. Our model is designed to weight more heavily the stats that are accumulated during the part of a game when the outcome is uncertain and to down-weight stats accumulated during the part of a game when the outcome appears to be determined – because, historically, what happens in low-leverage moments tells us less about a team’s true strength.
The final part of the formula for team strength deals with a team’s recent results. A team’s performance is seldom constant over the course of a whole season, so we don’t treat form as static. This means the model will be more impressed by what a team has done lately than what they did earlier in the season. A team who is on a run of good form is given a small “bonus” in SNR because, historically, hot teams have performed a bit better in their future games than cold teams have done. This is why every coach (and fan!) wants their team to “get hot at the right time” heading into end-of-year tournaments.
While I’m calling this a “hot streak” adjustment, it mostly helps account for things like shifts in team chemistry and injuries to key players. Take, for example, the 2022-23 season of Anadolu Efes (the Turkish pro club from Istanbul). Last year, Efes started the season without their star Shane Larkin, who missed several months with torn ligaments in his hand. As a result of Larkin’s absence, Efes stumbled their way to a 0.500-record (17-17) by the end of the 2022 calendar year. But with Larkin returning, Efes turned a corner in 2023, winning 14 of their last 16 games and capping the season off with a sweep of Pinar Karsiyaka to secure the Turkish Super League championship. Obviously, heading into the finals, we would have expected Efes to perform more like the red-hot team that finished the season than the ice-cold team who started it. And, because the model doesn’t directly incorporate information about player injuries (or expected playing time, in general), emphasizing recent form is one way to get a better sense for how a team is expected to perform in the next game.
The SNR metric is meant to estimate a team’s net rating or, basically, their expected margin of victory for a hypothetical 100-possession game. As such, the SNR model is informed by a team’s scoring margins over the course of the season. However, we are also applying the same approach to produce adjusted versions of other key team stats – like PPP, FG%, and TO rate – while accounting for the strength of the opponents faced and the other factors discussed in this post. So, for example, if a coach is preparing to play a team that has forced a lot of turnovers in their first few games of the season, the team adjusted stats would help the coach decide if the opponent was really a dominant defense or if, perhaps, they had simply played a soft slate of turnover-prone offenses that allowed them to “pad the stats”. Just like the SNR metric, these other team-adjusted stats help provide a clearer picture of a team’s true strengths and weaknesses. These adjustments will help coaches have realistic expectations about their own team and the teams they are preparing to play.
The development of the Synergy Net Rating metric was led by JP van Paridon. JP is building tools to help coaches, scouts, and players find winning team strategies as part of Synergy’s Actionable Analytics Team.